Smart Orchard Projects
Washington State's Tree Fruit Research Commission asked innov8.ag to lead a smart orchard project in collaboration with researchers from WSU & OSU at Chiawana Orchards. Our goal is to "sensorize" a orchard from multiple hardware providers, bringing together growers, data, and researchers to create a sustainable, "smart" orchard with insights that impact growers' bottom lines.
As we head into 2021 growing season, we've been commissioned to expand to a second Smart Orchard site, while also introducing our base crop count imaging capabilities to orchard & vineyard growers.
CLICK the buttons below for the respected Smart Orchard pages.
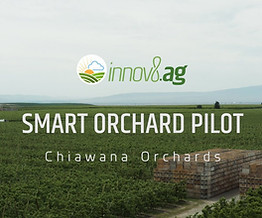
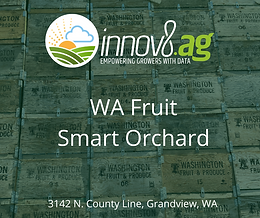
Our Approach
• Learn capabilities of modern orchard decision making with basic AI and Data Analytics
• Shift grower decision making process to enable management decisions based on unified data, and 'smart management'
• Help growers better manage their water usage, labor, equipment, and chemical usage
• Enable growers to better understand weather and climate change to make precise, informed decisions.
Our Goals
• Integrating data provided by a ecosystem sensors and data providers
• Provide consolidated data warehousing to collect & consolidate data, leveraging Microsoft Farmbeats for efficiencies thru API data interchanges
• Provide regular insights and analyses of integrated data
• Provide consolidated raw access to data
Data Sources:

Weather

Chemicals

Irrigation

Disease

Soil

Pests

Crop Health

Labor

Imagery
Research Publications:
Spatiotemporal water use mapping of a commercial apple orchard using UAS based spectral imagery
Abstract:
Crop water use estimation at high geospatial resolution is critical for site-specific irrigation management of perennial specialty crops. This study aims to map actual evapotranspiration (ETa) of a commercial apple orchard using unmanned aerial system (UAS) based thermal and multispectral imagery and a widely adopted METRIC (Mapping Evapotranspiration at High Resolution with Internalized calibration) energy balance model (UASM). Four imaging campaigns were conducted during the 2020 growth season and weather data for pertinent days was downloaded from the nearest WSU-AgWeatherNet network station. 24-h ET a was also calculated from the soil water balance (SWB) approach that used soil moisture data from sensors installed at three locations and down to depth of 111 cm. A high linear correlation (r) of 0.84 and non-significant difference (p = 0.5) was observed between UASM derived ET a (5.05 ± 0.8 [Mean ± Std. Dev.] mm day -1 ) and SWB calculated ET a (5.44 ± 1.81 mm day -1 ). Notable differences in spatiotemporal water use and crop-coefficients were observed within the orchard. A moderately strong correlation was also observed between the UASM derived crop-coefficients and multispectral imagery derived Normalized Difference Vegetation Index (r = 0.69) that may also be used for estimating actual crop water use. Overall, approach presented in this study may help identify under or over-irrigated areas within the orchard. It may also assist in developing site-specific irrigation prescription maps and schedules.
High spatiotemporal apple crop water use mapping using drone imagery
Abstract:
Evapotranspiration (ET) is the actual crop water use and its mapping at the tree level could be critical for identifying problem areas in the orchard and site-specific irrigation scheduling and management (Chandel et al., 2021). Conventionally, single point ET is estimated using generalized crop coefficients and weather data (Allen et al., 1998). Other ET estimation tools or approaches include soil water budget, soil moisture, canopy stomatal conductance, and eddy covariance flux measurements. However, those provide limited in-orchard spatial variability (Chandel et al., 2021). Satellite-based imagery with energy balance models can provide geospatial ET maps (Allen et al., 2007). These maps are limited due to spatiotemporal resolution (e.g. ~99 ft/pixel and 16 days for Landsat 7/8 satellites). Cloud cover is an additional challenge that leads to no or poor ET estimation using satellite-based imagery. Drone-based imagery is an alternative to offer on-demand crop ET characterization at high spatial resolution (up to mm/pixel). Such resolution is also useful to assess spatial crop water use variabilities and to focus on trees or specific rows. Therefore, our research team has successfully utilized and validated drone-based multispectral and thermal infrared imagery with the energy balance model for mapping geospatial ET of a modern apple orchard at 2.8 in/pixel.